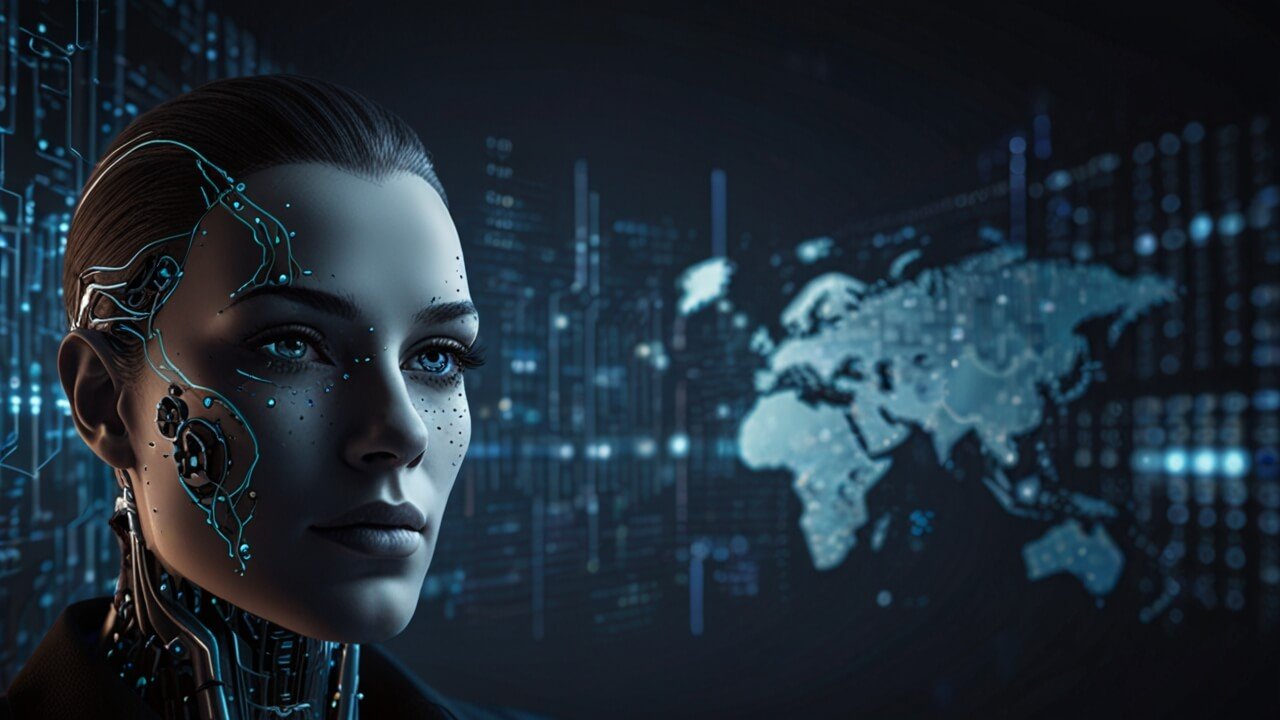
The Future of AIOps: Revolutionizing IT Operations with Artificial Intelligence
Artificial Intelligence for IT Operations (AIOps) is transforming how organizations manage their IT infrastructure by leveraging advanced AI and machine learning technologies to enhance operational efficiency and reliability. As the complexity of IT environments continues to grow, AIOps is emerging as a critical solution for addressing challenges related to system performance, incident management, and resource optimization.
This article explores the future of AIOps, examining emerging trends, evolving practices, and the impact of AI on IT operations. From predictive analytics and automation to the integration of multi-cloud environments and advanced security measures, the future of AIOps promises to reshape how organizations approach IT management and operational excellence.
Common Challenges in IT Operations Addressed by AIOps
1. Overwhelming Volume of Data
Problem: Modern IT environments generate vast amounts of data from various sources, including logs, metrics, and events. Managing and making sense of this data manually can be overwhelming and inefficient.
AIOps platforms will increasingly utilize advanced machine learning algorithms to analyze and correlate data from multiple sources. By automating data aggregation and analysis, AIOps can provide actionable insights, detect anomalies, and predict potential issues before they impact system performance.
2. Complex Incident Management
Problem: Identifying and resolving incidents in complex IT environments can be challenging, especially when multiple systems and applications are involved. Traditional incident management approaches may lack the speed and accuracy needed to address issues promptly.
Future AIOps solutions will leverage AI-driven incident management to automate detection, triage, and resolution of incidents. AI algorithms can analyze historical data, recognize patterns, and apply contextual information to expedite incident resolution, reducing downtime and minimizing impact.
3. Resource Optimization
Problem: Optimizing resource allocation and utilization across diverse IT environments can be difficult. Inefficient resource management can lead to increased costs and suboptimal performance.
AIOps platforms will employ AI-based optimization techniques to analyze resource usage patterns and make real-time adjustments. By predicting resource needs and automating scaling processes, AIOps can ensure efficient resource allocation, reduce costs, and improve overall system performance.
4. Proactive Performance Management
Problem: Ensuring consistent performance and availability of IT services requires proactive monitoring and management. Traditional approaches may lack the foresight needed to anticipate performance issues.
AIOps will focus on predictive performance management, using AI to analyze historical and real-time data to forecast potential performance bottlenecks. By identifying and addressing issues before they affect users, AIOps can enhance system reliability and user experience.
5. Integration with Multi-Cloud Environments
Problem: Managing IT operations across multiple cloud providers and on-premises systems presents integration and coordination challenges. Ensuring consistency and efficiency across diverse environments can be complex.
Future AIOps platforms will provide unified visibility and management capabilities across multi-cloud environments. By integrating data and insights from various cloud providers, AIOps can streamline operations, optimize resource usage, and ensure consistent performance across the entire IT ecosystem.
Emerging Trends in AIOps
1. Enhanced Predictive Analytics
Trend: The future of AIOps will see a greater emphasis on predictive analytics. Advanced AI algorithms will analyze historical data and current trends to forecast potential issues and performance degradation.
Impact: Enhanced predictive analytics will enable organizations to anticipate and address issues proactively, reducing downtime and improving system reliability. By identifying trends and anomalies before they escalate, AIOps can help organizations stay ahead of potential problems.
2. Increased Automation
Trend: Automation will play a central role in the future of AIOps, with AI-driven automation tools handling repetitive tasks such as incident detection, response, and resolution.
Impact: Increased automation will streamline IT operations, reduce manual intervention, and improve efficiency. Automated processes will enable faster incident resolution, better resource management, and more consistent performance.
3. Integration of AI and Machine Learning
Trend: The integration of AI and machine learning will become more advanced, with AIOps platforms utilizing sophisticated algorithms to analyze data, detect patterns, and optimize operations.
Impact: AI and machine learning will enhance the capabilities of AIOps by providing deeper insights into system behavior, automating decision-making processes, and improving overall operational efficiency.
4. Focus on User Experience
Trend: Future AIOps practices will place a stronger emphasis on user experience, aligning operational goals with the needs and expectations of end-users.
Impact: By prioritizing user experience, AIOps can ensure that IT operations are optimized for performance and reliability from the perspective of users. This focus will lead to improved service quality, higher user satisfaction, and better alignment with business objectives.
5. Advanced Security Measures
Trend: AIOps will increasingly incorporate advanced security measures, including AI-driven threat detection and response, to address emerging security challenges.
Impact: Enhanced security measures will help organizations identify and respond to security threats more effectively. AI-driven security capabilities will enable faster detection of vulnerabilities, automated incident response, and improved protection of IT assets.
6. Real-Time Insights and Visualization
Trend: The future of AIOps will feature advanced real-time insights and visualization tools, providing IT teams with comprehensive and actionable views of system performance.
Impact: Real-time insights and visualization will enable IT teams to monitor system health, detect issues quickly, and make informed decisions. Improved visibility will enhance operational efficiency and support proactive management of IT environments.
7. Seamless Integration with DevOps
Trend: AIOps will increasingly integrate with DevOps practices, fostering collaboration between development and operations teams to improve system reliability and performance.
Impact: The integration of AIOps with DevOps will streamline workflows, enhance communication, and drive continuous improvement. By aligning AIOps with DevOps principles, organizations can achieve better coordination and more efficient IT operations.
The Evolving Role of AIOps
1. From Reactive to Proactive
Evolution: AIOps is shifting from a reactive approach to a proactive one, using predictive analytics and automation to anticipate and address issues before they impact operations.
Impact: This shift will enable organizations to move from a reactive stance, where they respond to problems after they occur, to a proactive approach that prevents issues and optimizes performance. Proactive AIOps will lead to more reliable systems and improved operational efficiency.
2. Enhanced Collaboration Across Teams
Evolution: AIOps will facilitate enhanced collaboration between IT operations, development, and security teams. By providing a unified view of system performance and insights, AIOps will bridge gaps between different teams.
Impact: Improved collaboration will lead to better coordination, faster incident resolution, and more effective management of IT environments. Cross-functional teams will be able to work together more seamlessly, driving overall operational excellence.
3. Expansion of AIOps Use Cases
Evolution: The use cases for AIOps will expand beyond traditional IT operations to include areas such as application performance management, security operations, and business intelligence.
Impact: The expansion of AIOps use cases will provide organizations with a broader range of applications for AI-driven insights and automation. This will lead to more comprehensive and integrated management of IT and business operations.
4. AI-Driven Decision Making
Evolution: AIOps will increasingly focus on AI-driven decision-making, using machine learning algorithms to automate decisions and optimize operations.
Impact: AI-driven decision-making will enhance the accuracy and speed of operational decisions. By leveraging AI to analyze data and make decisions, organizations can improve efficiency, reduce errors, and achieve better outcomes.
5. Greater Emphasis on Data Privacy
Evolution: As data privacy regulations become more stringent, AIOps will place a greater emphasis on protecting sensitive data and ensuring compliance with privacy standards.
Impact: Enhanced data privacy measures will help organizations protect sensitive information and maintain compliance with regulatory requirements. AIOps platforms will incorporate features such as data encryption, access controls, and compliance monitoring to address privacy concerns.
6. Integration with Emerging Technologies
Evolution: AIOps will integrate with emerging technologies such as edge computing, blockchain, and IoT to enhance its capabilities and address new challenges.
Impact: The integration of AIOps with emerging technologies will provide organizations with new tools and insights for managing complex IT environments. By leveraging these technologies, AIOps can offer more comprehensive solutions and address evolving operational needs.
7. Focus on Business Outcomes
Evolution: The future of AIOps will involve a greater focus on aligning IT operations with business outcomes, ensuring that operational efforts contribute to organizational goals and success.
Impact: By focusing on business outcomes, AIOps will help organizations achieve strategic objectives and drive value from their IT investments. This alignment will lead to improved performance, better resource utilization, and enhanced overall success.
Real-World Examples of AIOps in Action
1. Case Study: IBM
IBM has leveraged AIOps to enhance its IT operations and deliver better services to its clients. By integrating AI-driven analytics and automation, IBM has improved incident management, optimized resource allocation, and enhanced overall operational efficiency.
2. Case Study: Splunk
Splunk utilizes AIOps to provide real-time insights and automation for IT operations. The company’s platform uses machine learning algorithms to analyze data, detect anomalies, and automate incident response, leading to improved performance and reliability.
3. Case Study: Microsoft Azure
Microsoft Azure employs AIOps to manage its cloud services and infrastructure. By using AI-driven monitoring and automation, Azure enhances system reliability, optimizes resource usage, and provides better performance for its customers.
4. Case Study: ServiceNow
ServiceNow integrates AIOps into its IT service management platform to improve incident management and automate workflows. The platform uses AI to analyze data, predict issues, and streamline operations, resulting in enhanced efficiency and service quality.
5. Case Study: Dynatrace
Dynatrace applies AIOps to provide advanced monitoring and performance management for its clients. By leveraging AI and machine learning, Dynatrace offers real-time insights, automated anomaly detection, and predictive analytics to enhance system reliability and user experience.
6. Case Study: Datadog
Datadog uses AIOps to deliver comprehensive monitoring and analytics for cloud environments. The company’s platform employs AI-driven algorithms to analyze data, detect anomalies, and optimize performance, helping organizations maintain reliable and efficient IT operations.
7. Case Study: New Relic
New Relic integrates AIOps to provide advanced application performance management and monitoring. By utilizing AI and machine learning, New Relic enhances its capabilities in incident detection, root cause analysis, and performance optimization.
Challenges and Considerations for the Future of AIOps
1. Integration with Existing Systems
Challenge: Integrating AIOps with existing IT systems and processes can be complex. Ensuring compatibility and seamless integration requires careful planning and execution.
Consideration: Organizations should develop a clear integration strategy, test AIOps solutions in a controlled environment, and work with vendors to ensure compatibility with existing systems and workflows.
2. Data Privacy and Security
Challenge: Managing data privacy and security within AIOps platforms is crucial, particularly as AI-driven solutions handle sensitive information.
Consideration: AIOps platforms should incorporate robust data privacy and security measures, including encryption, access controls, and compliance monitoring, to protect sensitive information and address regulatory requirements.
3. Skill Gaps and Training
Challenge: Implementing and managing AIOps solutions requires specialized skills and knowledge. Addressing skill gaps and providing adequate training can be a challenge.
Consideration: Organizations should invest in training and development programs for their IT teams, partner with AIOps vendors for support, and ensure that staff are equipped with the necessary skills to effectively utilize AIOps technologies.
4. Managing AI Bias and Fairness
Challenge: AI and machine learning algorithms may introduce bias or fairness issues if not carefully managed. Ensuring that AIOps solutions operate impartially and equitably is important.
Consideration: Organizations should implement practices for monitoring and addressing AI bias, conduct regular audits of AI models, and ensure that algorithms are designed to operate fairly and transparently.
5. Cost Management
Challenge: Implementing AIOps solutions can be costly, particularly for organizations with large and complex IT environments. Managing costs while realizing the benefits of AIOps requires careful planning.
Consideration: Organizations should evaluate the potential return on investment (ROI) of AIOps solutions, consider cost-benefit analyses, and explore options for scalable and cost-effective AIOps platforms.
6. Ensuring Accurate Data
Challenge: AIOps relies on accurate and high-quality data to provide meaningful insights and automation. Ensuring data accuracy and consistency is crucial for effective AIOps implementation.
Consideration: Organizations should implement data validation and cleansing processes, monitor data quality, and ensure that AIOps platforms have access to reliable and up-to-date information.
7. Handling Change Management
Challenge: Adopting AIOps solutions may require changes to existing processes and workflows. Managing change effectively is important for successful implementation.
Consideration: Organizations should develop a change management strategy, communicate changes to stakeholders, and provide support and guidance to teams during the transition to AIOps.
The Future Landscape of AIOps
1. Increased Adoption of AI and Machine Learning
The future of AIOps will see increased adoption of AI and machine learning technologies, enhancing the capabilities of AIOps platforms and providing more sophisticated insights and automation.
2. Greater Focus on Real-Time Analytics
AIOps will increasingly focus on real-time analytics, providing immediate insights and responses to operational events and performance metrics.
3. Expansion of Use Cases
The use cases for AIOps will expand beyond traditional IT operations to include areas such as security operations, business intelligence, and customer experience management.
4. Integration with Emerging Technologies
AIOps will integrate with emerging technologies such as edge computing, IoT, and blockchain, providing new capabilities and addressing evolving operational challenges.
5. Enhanced Collaboration and Communication
AIOps will facilitate better collaboration and communication between IT operations, development, and business teams, driving more effective and efficient management of IT environments.
6. Focus on Business Value
AIOps will place a greater emphasis on delivering business value, aligning IT operations with organizational goals and strategic objectives to drive success and achieve measurable outcomes.
7. Continuous Improvement and Innovation
The future of AIOps will be characterized by continuous improvement and innovation, with ongoing advancements in AI, machine learning, and automation driving enhanced capabilities and operational excellence.
Conclusion
The future of Artificial Intelligence for IT Operations (AIOps) is poised to revolutionize how organizations manage their IT infrastructure and operations. By addressing common challenges such as data volume, incident management, and resource optimization, and by embracing emerging trends such as predictive analytics, automation, and AI integration, AIOps is set to enhance operational efficiency and reliability.
As organizations prepare for the next wave of AIOps advancements, they will benefit from increased agility, improved performance, and better alignment with business objectives. The evolving landscape of AIOps promises to drive continuous improvement and innovation, positioning organizations for success in an increasingly complex and dynamic digital environment.