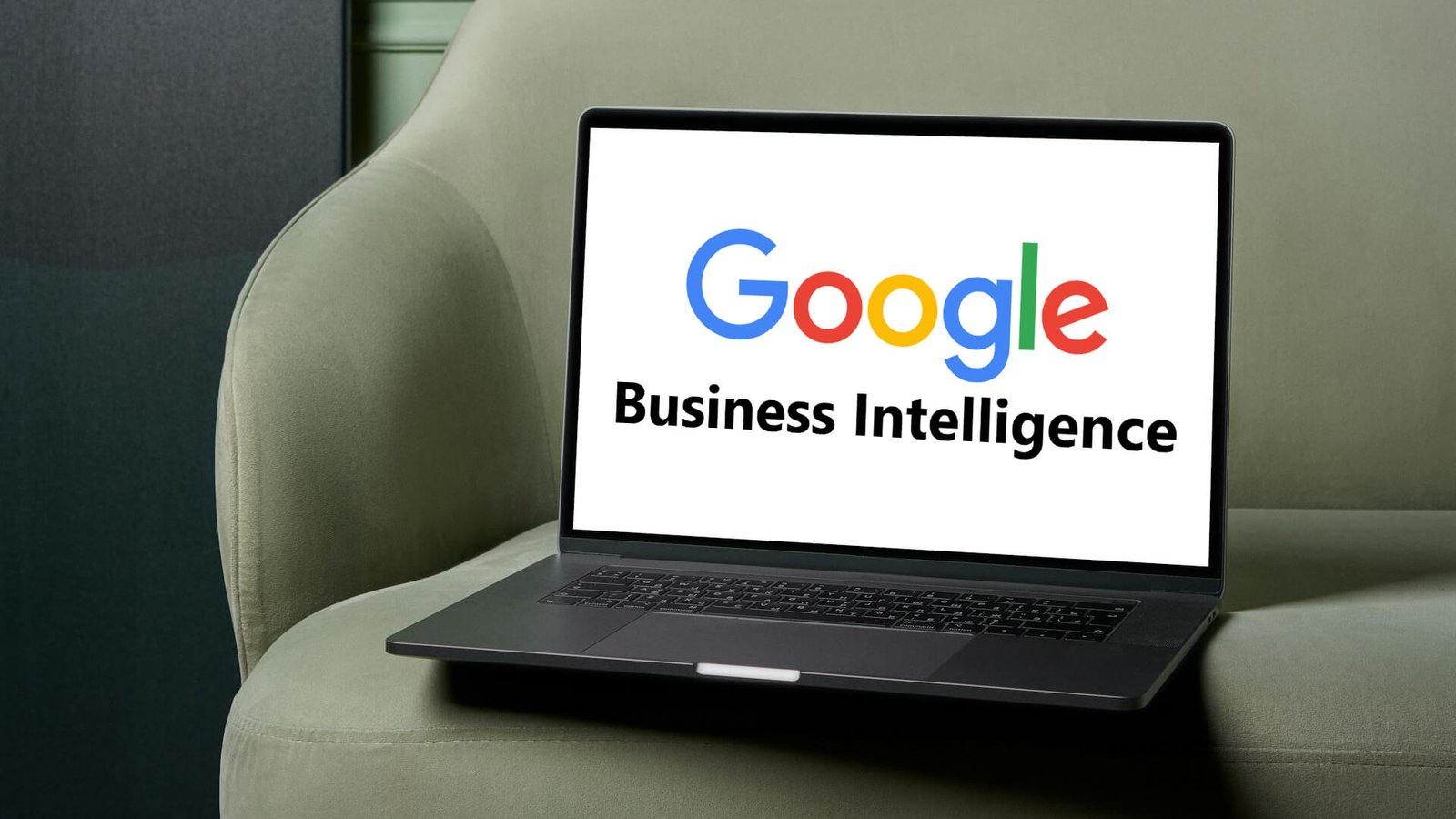
The Power of Google Business Intelligence: Revolutionizing Data-Driven Decision Making
Introduction: The New Era of Business Intelligence
In today’s hyper-connected world, data is often referred to as the new oil. Just as oil needs to be refined to be valuable, data must be analyzed and processed to deliver actionable insights. This transformation is where Business Intelligence (BI) plays a pivotal role, and Google’s suite of BI tools is at the forefront of this revolution.
By 2024, it is estimated that the total volume of data created and replicated globally will reach a staggering 180 zettabytes (Source: IDC). For context, this is equivalent to approximately 180 trillion gigabytes of data. This exponential increase in data underscores the urgent need for effective BI tools to manage, interpret, and utilize such vast quantities of information.
According to a report by Gartner, 87% of organizations claim to have adopted BI tools. However, only 37% of those users express satisfaction with their current BI solutions (Source: Gartner). This disparity often arises from the complexity and inefficiency of traditional BI tools, which Google’s innovative solutions aim to address.
Research by McKinsey & Company reveals that organizations driven by data are 23 times more likely to acquire customers, 6 times more likely to retain customers, and 19 times more likely to be profitable (Source: McKinsey & Company). This highlights the critical role of advanced BI tools in gaining a competitive advantage and making informed decisions.
This article delves into how Google’s Business Intelligence tools are transforming the landscape of data analysis and decision-making. We will explore the features, benefits, and best practices for utilizing Google’s BI solutions, offering a comprehensive guide to harnessing the power of data in your organization.
The Evolution of Business Intelligence
The concept of Business Intelligence has evolved significantly over the past few decades. Initially, BI was limited to basic reporting and querying tools, but advancements in technology and data volume have led to more sophisticated solutions.
Early Days of BI
In its early stages, BI primarily focused on static reports and basic data analysis. Tools were often rigid, with limited capabilities for handling large volumes of data or performing complex analyses. This era was characterized by:
- Manual Data Aggregation: Data was often collected manually, leading to time-consuming and error-prone processes.
- Static Reports: Reports were generated periodically and were not interactive, providing a snapshot rather than a dynamic view of the data.
- Limited Analytics: Analytical capabilities were basic, focusing on descriptive statistics rather than advanced analytics.
Modern BI Transformations
The advent of advanced technologies and the rise of big data have transformed BI into a more dynamic and integral part of business operations. Modern BI now includes:
- Advanced Analytics: Incorporating predictive and prescriptive analytics, which help organizations forecast future trends and make data-driven decisions.
- Interactive Dashboards: Providing real-time, interactive data visualizations that allow users to explore and analyze data dynamically.
- Self-Service BI: Empowering users to generate their own reports and insights without relying on IT departments.
Google’s BI tools are a key part of this transformation, leveraging cloud computing, machine learning, and real-time data processing to provide cutting-edge solutions for modern data analysis.
Key Components of Google Business Intelligence
Google offers a suite of BI tools designed to cater to various aspects of data analysis and visualization. Here’s a detailed look at the core components of Google’s BI ecosystem:
1. Google BigQuery
Google BigQuery is a powerful data warehouse solution that provides scalable and high-performance SQL querying capabilities.
Key Features
- Serverless Architecture: BigQuery eliminates the need for managing infrastructure, allowing you to focus on querying and analyzing data.
- Real-Time Data Processing: Supports real-time analytics, enabling immediate insights and decision-making.
- Advanced Machine Learning Integration: Integrates with Google’s machine learning tools to provide predictive analytics capabilities directly within the platform.
Best Practices for Using BigQuery
- Optimize Data Storage: Use efficient data storage formats and partition your data to enhance performance and reduce costs.
- Utilize Query Optimization Techniques: Implement best practices for writing SQL queries, such as using proper indexing and avoiding unnecessary data scans.
- Monitor and Manage Costs: Regularly review your BigQuery usage and set up budget alerts to prevent unexpected expenses.
2. Google Data Studio
Google Data Studio is a versatile data visualization tool that allows users to create interactive and customizable reports and dashboards.
Key Features
- Customizable Templates: Offers a range of templates that can be tailored to meet specific reporting needs.
- Real-Time Collaboration: Supports real-time collaboration, allowing multiple users to work on reports simultaneously.
- Data Source Integration: Connects to a wide variety of data sources, including Google Analytics, Google Sheets, and external databases.
Best Practices for Using Data Studio
- Design Engaging Reports: Focus on creating visually appealing and easy-to-understand reports that convey insights effectively.
- Implement Interactive Elements: Add filters, controls, and drill-down capabilities to allow users to interact with the data.
- Regularly Update Reports: Ensure that reports and dashboards are updated regularly to reflect the latest data.
3. Google Looker
Google Looker is a modern BI platform designed for advanced data exploration and visualization.
Key Features
- Data Exploration and Ad-Hoc Analysis: Allows users to perform ad-hoc analysis and explore data interactively without needing complex queries.
- Customizable Dashboards: Create dashboards that are tailored to specific business needs and user roles.
- LookML Data Modeling: Use LookML to define and manage data relationships and calculations centrally.
Best Practices for Using Looker
- Develop Comprehensive Data Models: Use LookML to create reusable and maintainable data models that serve various analytical needs.
- Promote Data Literacy: Encourage users to leverage Looker’s data exploration capabilities to enhance their analytical skills.
- Foster Collaboration: Share insights and dashboards with team members to support collaborative decision-making.
Integrating Google BI Tools into Your Workflow
Successful integration of Google’s BI tools into your workflow can lead to more effective data management and decision-making. Here are some strategies for seamless integration:
1. Streamline Data Ingestion
Efficient data ingestion is crucial for timely analysis. Utilize Google Cloud’s data transfer services, such as:
- Data Transfer Service: Automates the process of loading data from external sources into BigQuery.
- Cloud Storage: Store and manage large volumes of data in Google Cloud Storage before analyzing it with BigQuery.
Best Practices
- Automate Data Pipelines: Set up automated data pipelines to ensure that data is ingested regularly and consistently.
- Validate Data Quality: Implement data validation checks to ensure that the data being ingested is accurate and complete.
2. Establish a Data Governance Framework
A robust data governance framework is essential for maintaining data quality, consistency, and security. Key components include:
- Data Ownership: Define clear ownership and stewardship roles for different data assets.
- Data Quality Standards: Establish standards for data accuracy, completeness, and consistency.
- Access Controls: Implement access controls to protect sensitive data and ensure that only authorized users can access specific datasets.
Best Practices
- Document Data Policies: Create and maintain documentation for data governance policies and procedures.
- Regularly Review Access Permissions: Periodically review and update access permissions to align with changing business needs and user roles.
3. Promote Data Literacy
Enhancing data literacy across your organization can empower employees to make better data-driven decisions. Consider:
- Training Programs: Invest in training programs to improve employees’ skills in using Google BI tools and interpreting data.
- Support Resources: Provide access to support resources, such as online tutorials, user communities, and help desks.
Best Practices
- Encourage Continuous Learning: Foster a culture of continuous learning and curiosity about data.
- Provide Hands-On Experience: Offer opportunities for employees to gain practical experience with BI tools through workshops and projects.
Real-World Applications and Case Studies
Exploring real-world applications and case studies can provide valuable insights into how Google’s BI tools are used in various industries.
1. Retail Industry
A prominent retailer leveraged Google BigQuery to enhance their inventory management and customer targeting. By analyzing customer purchase data in real-time, they were able to:
- Optimize Inventory Levels: Predict demand patterns and adjust inventory levels accordingly, reducing stockouts and overstock situations.
- Enhance Customer Segmentation: Identify customer preferences and tailor marketing campaigns to specific segments, improving conversion rates.
Outcome
- Increased Sales: The retailer saw a significant increase in sales due to better-targeted promotions and improved inventory management.
- Improved Efficiency: Streamlined inventory processes, resulting in cost savings and operational efficiency.
2. Healthcare Sector
A healthcare provider used Google Looker to analyze patient data and improve operational efficiency. Key applications included:
- Patient Care Analysis: Monitor patient care trends and identify areas for improvement in service delivery.
- Operational Insights: Analyze administrative processes to streamline operations and reduce costs.
Outcome
- Enhanced Patient Outcomes: Improved patient care through data-driven insights into treatment effectiveness and care quality.
- Cost Savings: Reduced operational costs by optimizing administrative processes and resource allocation.
3. Financial Services
A financial institution utilized Google’s BI tools to enhance fraud detection and risk management. Key initiatives included:
- Fraud Detection: Analyze transactional data to identify and prevent fraudulent activities in real-time.
- Risk Management: Monitor and manage financial risks through advanced analytics and predictive modeling.
Outcome
- Reduced Fraudulent Activities: Significantly decreased instances of fraud by leveraging real-time analytics and machine learning models.
- Improved Risk Management: Enhanced ability to predict and mitigate financial risks, leading to more stable financial performance.
Conclusion
As organizations continue to grapple with an ever-increasing volume of data, leveraging advanced Business Intelligence tools becomes increasingly crucial. Google’s BI solutions—BigQuery, Data Studio, and Looker—offer powerful capabilities for transforming data into actionable insights and driving strategic decision-making.
By adopting these tools and implementing best practices, you can unlock the full potential of your data, gain a competitive edge, and achieve better business outcomes. Embrace the power of Google BI, and position your organization for success in the data-driven future.
Sources
IDC, “Data Age 2025: The Evolution of Data to Everything,” https://www.idc.com |
Gartner, “Magic Quadrant for Analytics and Business Intelligence Platforms,” https://www.gartner.com |
McKinsey & Company, “The Age of Analytics: Competing in a Data-Driven World,” https://www.mckinsey.com |